2017-Aug-17
If uncertainties encode bet preferences as represented by probabilities,
Bayesianism is a collection of Dutch book arguments proving that probabilities
must be consistent with each other (defining a probability measure) to be
rational.
Weisberg has an excellent paper that explains the details.
On the other hand, the Fundamental Theorem of Asset Pricing proves that for
prices to be arbitrage-free, they must be conditional expectations.
Details on the relevant results are found in
“The Mathematics of Arbitrage”, by Delbaen and Schachermayer.
Having a consistent probability function has been shown to be
equivalent to minimizing a proper scoring rule.
And conditional expectations have been shown to minimize
Bregman divergences.
Et cetera.
The correspondance between these theories is alluded to by Nau.
2017-Jun-28
The 700MHz (2014) and 2500MHz (2015) spectrum auctions generated revenues of
5,270,636,002 CAD from 302 licenses and 755,371,001 CAD from 97 licenses.
Both
auctions used a combinatorial clock auction (CCA) format involving an
ascending clock phase followed by a sealed-bid supplementary stage where
bids could be made on packages of products.
Final prices were determined
using Vickrey pricing with a core-adjustment. An activity
rule was used which required bidders to make bids or lose eligibility to
bid in later clock rounds, along with a revealed preference rule which
allows the eligibility limit to be exceeded as long as consistency
checks are satisfied. For full details on the auction formats see the
official documentation
(700MHz rules,
700MHz additional details,
2500MHz rules);
and the record of bids placed is here
for 700MHz and
here
for 2500MHz.
Bid consistency
The revealed preference rule prevents some inconsistent behavior but not all.
By “truthful”, we mean bids that are true indications of subjective value,
and by “consistent” we mean bids that are indications of some fixed set of
valuations, possibly not the bidder’s actual valuations.
The following table gives the values of Afriat’s critical cost
efficiency index (CCEI) for the 700MHz auction. Recall
that for a CCEI value \(x\), if \(x < 1\) there is at least some
intransitivity in preferences (i.e. inconsistent bidding) and \(1-x\) can be
interpreted as the fraction of expenditure wasted making inefficient
choices (see this
by S. Kariv for more).
Bidder |
CCEI (clock rounds) |
CCEI (clock and supp. rounds) |
Bell |
0.930 |
0.417 |
Bragg |
0.880 |
0.420 |
Feenix |
1 |
1 |
MTS |
0.996 |
0.627 |
Novus |
1 |
1 |
Rogers |
0.998 |
0.742 |
SaskTel |
1 |
1 |
TBayTel |
1 |
1 |
Telus |
0.970 |
0.488 |
Videotron |
0.879 |
0.560 |
Kroemer et al.
conclude for the 700MHz auction, “the
numbers suggest that bidders deviated substantially from straightforward
bidding” in the clock rounds. But “it is not unreasonable to believe
that bidders tried to bid up to their true valuation in the
supplementary stage” because of higher bid amounts compared to the clock
rounds.
The next table gives CCEI values for the 2500MHz
auction. We extend the definition of CCEI to apply to supplementary bids
as in Kroemer’s paper.
Bidder |
CCEI (clock rounds) |
CCEI (clock and supp. rounds) |
Bell |
0.913 |
0.712 |
Bragg |
0.920 |
0.530 |
Corridor |
1 |
1 |
MTS |
1 |
1 |
Rogers |
1 |
1 |
SSi Micro |
1 |
1 |
TBayTel |
1 |
1 |
Telus |
0.997 |
0.996 |
Videotron |
1 |
1 |
WIND |
1 |
1 |
Xplornet |
1 |
0.578 |
Kroemer et al. (Sec. 5.2) also point out that the
total number of bids submitted in the 700MHz auction was much smaller
than the number of possible bids, which probably indicates untruthful
bidding since an omitted
package must have valuation less than or equal to its (low) opening price.
The same observation holds for the 2500MHz auction. More exactly, the
auction formats enforced a limit on the number of packages bidders were
allowed to submit, which was in the hundreds, and bidders generally did
not reach the limit.
Ideally, we would determine whether the bids made are consistent with a
non-truthful strategy incorporating gaming and/or coordination.
The papers
Janssen and Karamychev -
“Raising Rivals’ Cost in Multi-unit Auctions”
and
Janssen and Kasberger -
“On the Clock of the Combinatorial Auction”
derive Bayesian Nash equilibria under gaming preferences and conclude that
GARP is not violated in equilibrium gaming strategies.
We note that the assumptions in these game models do not include all features
of the CCAs under consideration e.g.
discrete products, many bidders, public aggregate excess
demand, revealed preference rule, initial EP limit,
supplementary package limit, 50% mid-auction deposits.
Bids, budgets, and final prices
Bidders may have a notion of a budget – the maximum they are willing to
spend. But how should this correspond to the maximum they should bid? In
general, bidders may end up paying the exact amount of their highest
bid, but looking at the data we see bid prices and final prices can be
very different in practice.
The following tables show figures from both auctions that illustrate this
difference.
All prices are given in CAD.
700MHz auction: highest bid placed.
Average ratio: 0.192. Max ratio: 0.766.
Bidder |
Max bid (\(M\)) |
Allocation stage final price (\(p\)) |
Ratio (\(p/M\)) |
Final clock bid |
Bell |
3,999,999,000 |
565,705,517 |
0.141 |
1,366,867,000 |
Bragg |
141,894,000 |
20,298,000 |
0.143 |
38,814,000 |
Feenix |
60,650,000 |
284,000 |
0.005 |
346,000 |
MTS |
73,067,000 |
8,772,072 |
0.120 |
10,853,000 |
Novus |
112,359,000 |
0 |
0 |
0 |
Rogers |
4,299,949,000 |
3,291,738,000 |
0.766 |
3,931,268,000 |
Sasktel |
75,000,000 |
7,556,929 |
0.101 |
11,927,000 |
TbayTel |
7,683,000 |
0 |
0 |
0 |
Telus |
3,750,000,000 |
1,142,953,484 |
0.305 |
1,313,035,000 |
Videotron |
677,524,000 |
233,328,000 |
0.344 |
468,530,000 |
700MHz auction: (highest) bid placed on package eventually won.
Average ratio: 0.447. Max ratio: 0.766.
Bidder |
Max bid on won package (\(W\)) |
Allocation stage final price (\(p\)) |
Ratio (\(p/W\)) |
Allocation stage Vickrey price |
Bell |
2,583,868,000 |
565,705,517 |
0.219 |
565,705,000 |
Bragg |
51,000,000 |
20,298,000 |
0.398 |
20,298,000 |
Feenix |
425,000 |
284,000 |
0.668 |
284,000 |
MTS |
40,000,000 |
8,772,072 |
0.219 |
3,198,000 |
Novus |
N/A |
0 |
N/A |
0 |
Rogers |
4,299,949,000 |
3,291,738,000 |
0.766 |
3,291,738,000 |
Sasktel |
62,400,000 |
7,556,929 |
0.121 |
2,755,000 |
TbayTel |
N/A |
0 |
N/A |
0 |
Telus |
1,607,300,000 |
1,142,953,484 |
0.711 |
1,142,953,000 |
Videotron |
490,000,000 |
233,328,000 |
0.476 |
233,328,000 |
2500MHz auction: highest bid placed.
Average ratio: 0.135. Max ratio: 0.277.
Bidder |
Max bid (\(M\)) |
Allocation stage final price (\(p\)) |
Ratio (\(p/M\)) |
Final clock bid |
Bell |
542,746,000 |
28,730,000 |
0.053 |
76,214,000 |
Bragg |
35,935,000 |
4,821,021 |
0.134 |
12,091,000 |
Corridor |
9,300,000 |
2,299,000 |
0.247 |
N/A |
MTS |
13,609,000 |
2,242,000 |
0.165 |
2,609,000 |
Rogers |
304,109,000 |
24,049,546 |
0.079 |
52,343,000 |
SSi Micro |
851,000 |
0 |
0 |
0 |
TBayTel |
12,001,000 |
1,731,000 |
0.144 |
1,731,000 |
Telus |
1,771,723,000 |
478,819,000 |
0.270 |
1,038,472,000 |
Videotron |
749,128,000 |
66,552,980 |
0.089 |
231,851,000 |
WIND |
22,609,000 |
0 |
0 |
0 |
Xplornet |
91,974,000 |
25,472,454 |
0.277 |
57,839,000 |
2500MHz auction: (highest) bid placed on package eventually won.
Average ratio: 0.235. Max ratio: 0.410.
Bidder |
Max bid on won package (\(W\)) |
Allocation stage final price (\(p\)) |
Ratio (\(p/W\)) |
Allocation stage Vickrey price |
Bell |
536,563,000 |
28,730,000 |
0.054 |
28,730,000 |
Bragg |
19,000,000 |
4,821,021 |
0.254 |
3,536,000 |
Corridor |
6,440,000 |
2,299,000 |
0.357 |
2,299,000 |
MTS |
11,000,000 |
2,242,000 |
0.204 |
2,242,000 |
Rogers |
OR |
24,049,546 |
N/A |
21,252,000 |
SSi Micro |
N/A |
0 |
N/A |
0 |
TBayTel |
12,001,000 |
1,731,000 |
0.144 |
1,731,000 |
Telus |
1,771,723,000 |
478,819,000 |
0.270 |
478,819,000 |
Videotron |
358,477,000 |
66,552,980 |
0.186 |
61,092,000 |
WIND |
N/A |
0 |
N/A |
0 |
Xplornet |
62,200,000 |
25,472,454 |
0.410 |
22,917,000 |
Across both auctions, we see that bidders paid an average of 16% of
their maximum bid placed, where each bidder is equally weighted.
Misc. notes
Researchers design approximation algorithms for winner and price
determination in auctions (in e.g. this paper)
because exact
optimization can be intractable as the number of bids grows, at least in
the worst case. However, in these recent auction instances, theoretical
intractability did not present a problem because the solution was
computable in a small amount of time.
The 2500MHz allocation stage involved 2,239 bids and a
GLPK-powered solver finds the winners and final prices in a couple
minutes on a standard computer.
Simulations involving well over 30,000 random bids still take a feasible amount
of time.
In the 2500MHz auction, there were 4 pairs of package submissions where
the lower-priced package had strictly higher quantities of products.
In this case the lower-priced package is superfluous.
This table shows the packages, submitted by Bell.
|
Price of larger package (CAD) |
Price of smaller package (CAD) |
Number of products difference |
1 |
535,917,000 |
536,214,000 |
3 |
2 |
536,628,000 |
536,645,000 |
3 |
3 |
536,401,000 |
536,545,000 |
3 |
4 |
536,434,000 |
536,563,000 |
3 |
Acknowledgement: Thanks to Z. Gao for pointers.
2017-Jun-21
http://www.anagram.com/jcrap/
The prestigious Journal of Craptology is an open-access publication in the
area of cryptology.
2017-Jun-9
(a partial list)
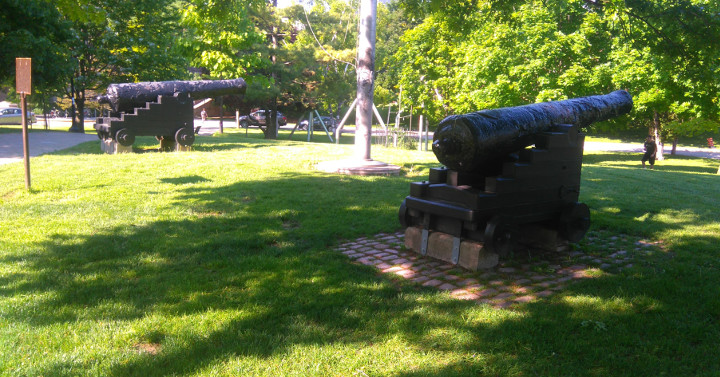
(Above: U of T cannons pointed at Ryerson)
noise |
low |
power plugs |
yes |
network access |
good |
ergonomics |
poor |
busyness |
busy |
typical hours |
8:30-11 |
Richard Charles Lee Canada-Hong Kong Library
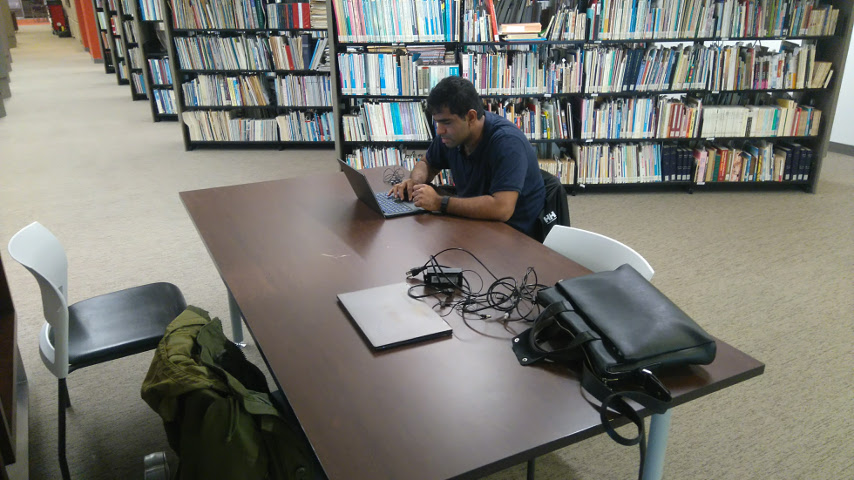
noise |
low |
power plugs |
yes |
network access |
good |
ergonomics |
poor |
busyness |
modest |
typical hours |
10-7 |
noise |
low |
power plugs |
some |
network access |
poor |
ergonomics |
poor |
busyness |
busy |
typical hours |
8:30-11 |
noise |
medium |
power plugs |
yes |
network access |
poor |
ergonomics |
ok |
busyness |
busy |
typical hours |
9-6 |
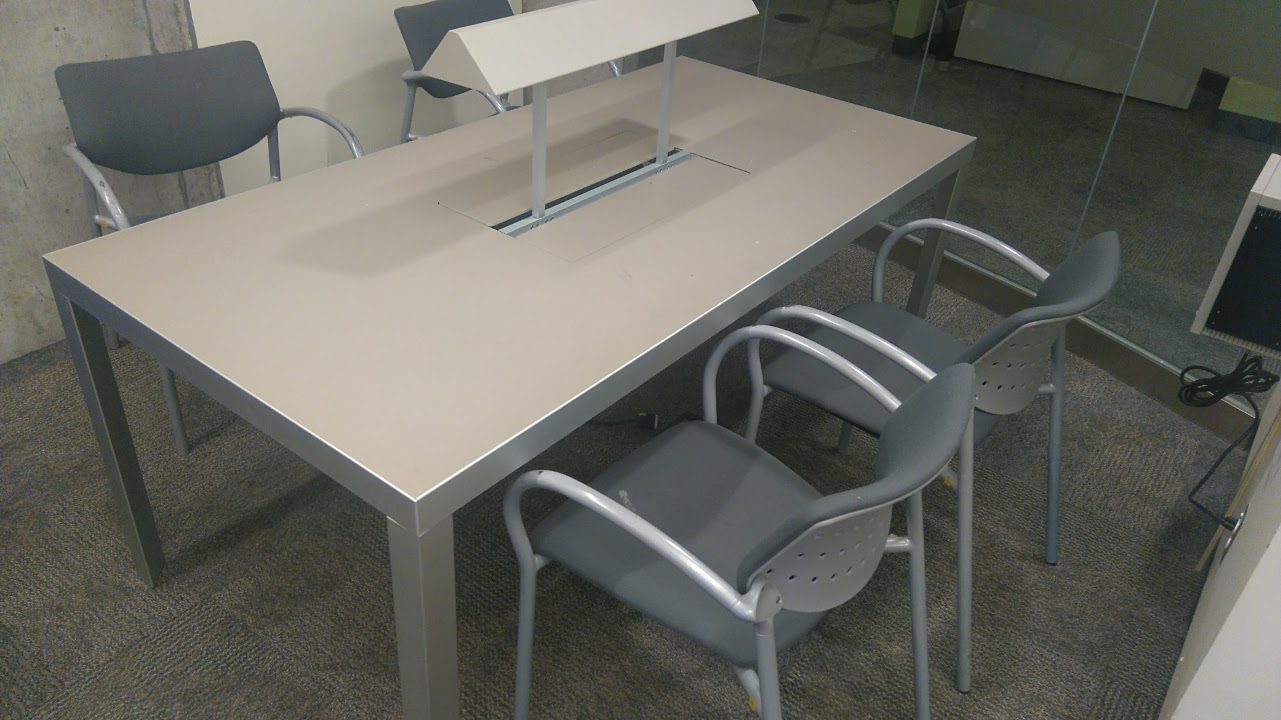
noise |
low |
power plugs |
some |
network access |
ok |
ergonomics |
poor |
busyness |
busy |
typical hours |
9-5 |
noise |
low |
power plugs |
some |
network access |
? |
ergonomics |
poor |
busyness |
busy |
typical hours |
8:30-11:45 |
noise |
low |
power plugs |
yes |
network access |
? |
ergonomics |
poor |
busyness |
busy |
typical hours |
9-9 |
noise |
? |
power plugs |
yes |
network access |
good |
ergonomics |
poor |
busyness |
? |
typical hours |
8:30-11:30 |
noise |
? |
power plugs |
yes |
network access |
good |
ergonomics |
poor |
busyness |
? |
typical hours |
9:30-9:30 |
East Asian Library
noise |
? |
power plugs |
no |
network access |
? |
ergonomics |
poor |
busyness |
? |
typical hours |
9-7:30 |
noise |
? |
power plugs |
no |
network access |
? |
ergonomics |
poor |
busyness |
? |
typical hours |
9-5 |
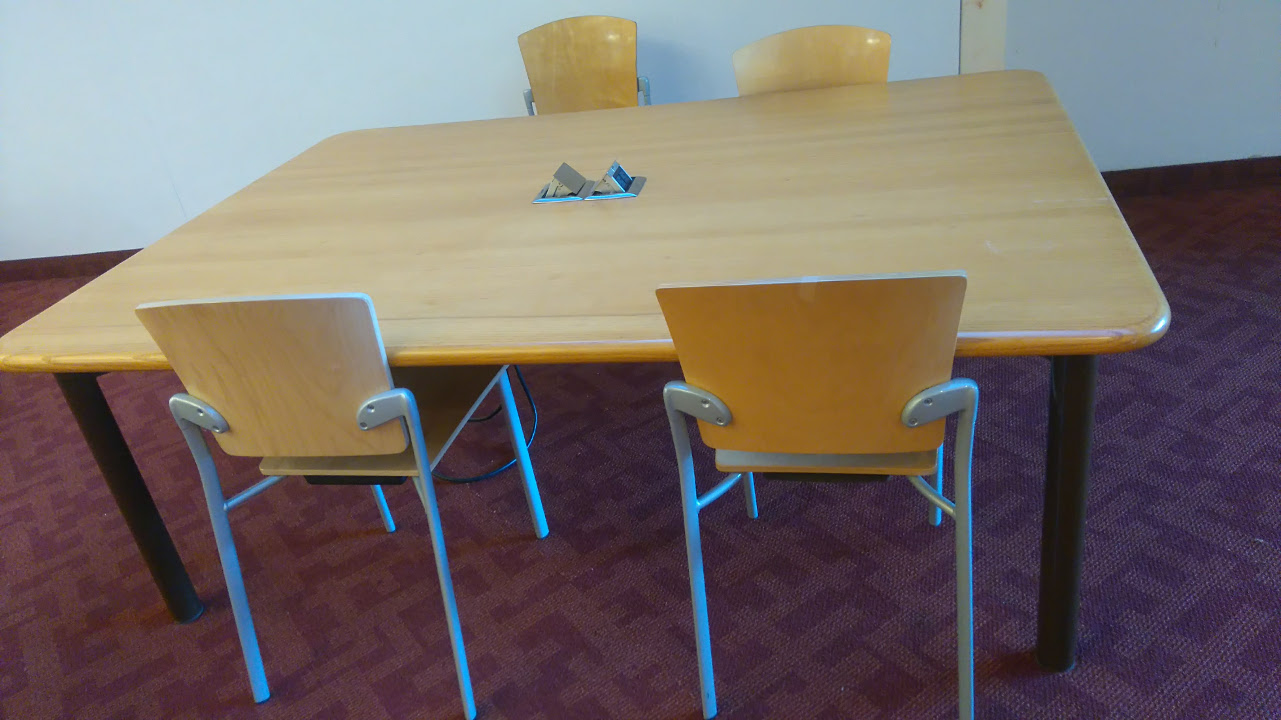
noise |
low |
power plugs |
some |
network access |
yes |
ergonomics |
poor |
busyness |
medium |
typical hours |
9-8:30 |
noise |
low |
power plugs |
some |
network access |
good |
ergonomics |
poor |
busyness |
medium |
typical hours |
9-8:30 |
Other areas with seating
noise |
moderate |
power plugs |
yes |
network access |
ok |
ergonomics |
poor |
busyness |
busy |
typical hours |
? |
2017-May-27
Let’s use python to train a Markov chain generator using all the tweets from a
certain list of users, say
this one.
We’ll use the following libraries.
from functional import seq
import markovify
import re
import tweepy
import unidecode
To use the Twitter API, we need to authenticate ourselves.
Register for your personal keys at https://apps.twitter.com/ and then create
a config.json
file that looks like this
{
"consumer_key": "...",
"consumer_secret": "...",
"access_key": "...",
"access_secret": "..."
}
Now we can initialize the Twitter API provided by tweepy.
config = seq.json('config.json').dict()
auth = tweepy.OAuthHandler(
config['consumer_key'], config['consumer_secret'])
auth.set_access_token(config['access_key'], config['access_secret'])
api = tweepy.API(auth)
First we write the following function (based on this gist)
which returns the most recent tweets of a given user.
The API limits us to at most 3240 tweets per user.
def get_user_tweets(screen_name):
alltweets = []
# 200 is the maximum allowed count
# 'extended' means return full unabridged tweet contents
new_tweets = api.user_timeline(screen_name=screen_name, count=200,
tweet_mode='extended')
alltweets.extend(new_tweets)
# save the id of the oldest tweet less one
oldest_id = alltweets[-1].id - 1
# keep grabbing tweets until there are no tweets left to grab
while len(new_tweets) > 0:
# since we're grabbing 200 at a time, we use `max_id` to
# ask for a certain range of tweets
new_tweets = api.user_timeline(
screen_name = screen_name, count=200,
tweet_mode='extended', max_id=oldest_id)
alltweets.extend(new_tweets)
#update the id of the oldest tweet less one
oldest_id = alltweets[-1].id - 1
print("...{} tweets downloaded so far".format(len(alltweets)))
# put each tweet on a single line
tweet_texts = [re.sub(r'\s*\n+\s*', ' ', tweet.full_text)
for tweet in alltweets]
return tweet_texts
The other interaction with Twitter we need to perform is get all users in a
list.
We’ll write a function that fetches the usernames and calls get_user_tweets
on each:
def get_list_tweets(screen_name, list_name):
'''
params: `screen_name` is the username of the owner of the list,
`list_name` is the name of the list found in the URL
'''
# get list of all users in list
user_names = []
for user in tweepy.Cursor(
api.list_members,
screen_name,
list_name).items():
user_names.append(user.screen_name)
# for each user, get their tweets
list_tweets = []
for user_name in user_names:
list_tweets += get_user_tweets(user_name)
print('Found {1} tweets from @{2}.'
.format(len(list_tweets), user_name))
return list_tweets
Let’s run get_list_tweets
and save the output to a file.
tweets = get_list_tweets('Grognor', 'weird-sun-twitter')
with open('data/tweetdump.txt', 'w') as f:
f.write('\n'.join(tweets))
With all of the raw data saved, we’re done with the Twitter API and we can
process the data and auto-generate tweets offline.
Assuming the file tweetdump.txt
has a set of tweets, one per line, we
load them as a list of strings tweets
.
tweets = open('data/tweetdump.txt').readlines()
Some processing needs to be done in order to get high quality text from the
tweets.
The next function process_tweet
is called on each one.
def process_tweet(tweet):
# convert to ASCII
tweet = unidecode.unidecode(tweet)
# remove URLs
tweet = re.sub(r'http\S+', '', tweet)
# remove mentions
tweet = re.sub(r'@\S+', '', tweet)
tweet = tweet.strip()
# append terminal punctuation if absent
if len(tweet) > 0:
last_char = tweet[-1]
if last_char not in '.!?':
tweet += '.'
return tweet
processed_tweets = [ process_tweet(tweet) for tweet in tweets ]
And we remove any tweets that aren’t useful.
def is_excluded(tweet):
ex = False
# no RTs
ex = ex or bool(re.match(r'^RT', tweet))
# remove whitespace-only tweets
ex = ex or bool(re.match(r'^\s*$', tweet))
return ex
good_tweets = [ tweet for tweet in processed_tweets
if not is_excluded(tweet) ]
We save the fully processed tweets for easy access later.
with open('data/processed_tweets.txt', 'w') as f:
f.write('\n'.join(good_tweets))
The markovify
library lets us train, and generate from, a Markov chain very
easily.
Just load the training text and set a state size.
text = open('data/processed_tweets.txt').read()
text_model = markovify.Text(text, state_size=3)
for x in range(5):
print('* ' + text_model.make_short_sentence(140))
Some favorites:
- “It is no coincidence we call them gods because we suppose they are trying to
convince Robin Hanson.”
- “Tell anyone who does not produce Scott Alexander.”
- “Weird sun is a costly signal of the ability to remember sources of
information, not just the study of complex manifolds.”
- “If you read The Hobbit backwards, it’s about a layer of radioactive ash that
develops the capacity to become larger.”
- “When you read a physical book, you get a dust speck in the eye.”
- “We all continuously scream about how the people in it are breaking the
awkward silence.”
- “People are important, but so are lexicographic preferences.”
- “You don’t need an expert Bayesian Epistemologist to ensure it’s not a markov
chain.”
2017-May-20
ShellJS is a JS library that provides
functions like cd()
and ls()
which you can use to write Node scripts
instead of bash scripts.
That’s great for scripts, but what about an interactive shell?
Well, we could just run the Node repl and import ShellJS:
$ node
> require('shelljs/global');
{}
> pwd()
{ [String: '/tmp']
stdout: '/tmp',
stderr: null,
code: 0,
cat: [Function: bound ],
exec: [Function: bound ],
grep: [Function: bound ],
head: [Function: bound ],
sed: [Function: bound ],
sort: [Function: bound ],
tail: [Function: bound ],
to: [Function: bound ],
toEnd: [Function: bound ],
uniq: [Function: bound ] }
Hmm, that’s a little verbose, and we might want to avoid manually importing
ShellJS.
We also might want more features than the Node repl offers, such as vi
keybindings.
We can get vi keybindings with rlwrap, but then tab completion goes away.
The solution is given in this SO answer.
First we need to install an rlwrap filter that negotiates tab-completion with a
Node repl.
The filter file can be found at that link, where it’s called node_complete
.
Put node_complete
in $RLWRAP_FILTERDIR
, which should be the folder on your
system containing the RlwrapFilter.pm
Perl module.
For me it’s /usr/share/rlwrap/filters
.
Now rlwrap is ready to negotiate tab completion, but the Node repl isn’t.
We’ll have to actually write our own Node repl, which is easy because the
repl
module gives us all the tools we need.
We’ll create a file called, say, myrepl.js
, the contents of which are also
given in the SO answer, only 9
lines.
This script starts a repl with a hook to negotiate tab completion with rlwrap.
If myrepl.js
is in ~/bin
, now we can run
$ rlwrap -z node_complete -e '' -c ~/bin/myrepl.js
and have both JS tab completion and rlwrap features, such as vi keybindings
if that’s what we’ve configured.
Let’s create a file called mysh
with the following contents:
#!/usr/bin/env bash
rlwrap -z node_complete -e '' -c ~/bin/myrepl.js
Assuming ~/bin
is in our path variable, we can put mysh
there and launch
our shell anywhere by just running mysh
.
So far so good but we wanted to automatically import ShellJS.
In myrepl.js
, add the following:
var shell = require('shelljs');
Object.assign(myrepl.context, shell);
Those two lines add all the ShellJS functions to the JS global object inside
the repl.
We have:
$ mysh
> pwd()
{ [String: '/tmp']
stdout: '/tmp',
stderr: null,
code: 0,
cat: [Function: bound ],
exec: [Function: bound ],
grep: [Function: bound ],
head: [Function: bound ],
sed: [Function: bound ],
sort: [Function: bound ],
tail: [Function: bound ],
to: [Function: bound ],
toEnd: [Function: bound ],
uniq: [Function: bound ] }
Progress.
Now, how do we clean up this output?
The repl
module allows us to define a custom writer
.
This is a function which takes the
output of a line of JS and returns a string to represent the output in the
repl.
What we need to do is intercept objects like the one returned by pwd()
above
and only show the stderr
and stdout
properties.
Add the following near the beginning of myrepl.js
:
var util = require('util');
var myWriter = function(output) {
var isSS = (
output &&
output.hasOwnProperty('stdout') &&
output.hasOwnProperty('stderr'));
if (isSS) {
var stderrPart = output.stderr || '';
var stdoutPart = output.stdout || '';
return stderrPart + stdoutPart;
} else {
return util.inspect(output, null, null, true);
}
};
And load this writer by changing
var myrepl = require("repl").start({terminal:false});
to
var myrepl = require("repl").start({
terminal: false,
writer: myWriter});
Now we get
Much better.
However, since the echo
function prints its argument to the console and
returns an object with it in the stdout
property, we get this:
$ mysh
> echo('hi')
hi
hi
I haven’t solved this issue quite yet although I’d be surprised if there isn’t
a reasonable solution out there.
You can add to mysh
and myrepl.js
to get more features, such as colors,
custom evaluation, custom pretty printing, other pre-loaded libraries, et
cetera.
The sky is the limit.
I added an inspect
function which allows us to see the full ShellJS output
of a command if we really want it.
My complete myrepl.js
file is:
#!/usr/bin/env node
var util = require('util');
var colors = require('colors/safe');
var inspect = function(obj) {
if (obj && typeof obj === 'object') {
obj['__inspect'] = true;
}
return obj;
};
var myWriter = function(output) {
var isSS = (
output &&
output.hasOwnProperty('stdout') &&
output.hasOwnProperty('stderr') &&
!output.hasOwnProperty('__inspect'));
if (isSS) {
var stderrPart = output.stderr || '';
var stdoutPart = output.stdout || '';
return colors.cyan(stderrPart + stdoutPart);
} else {
if (typeof output === 'object') {
delete output['__inspect'];
}
return util.inspect(output, null, null, true);
}
};
// terminal:false disables readline (just like env NODE_NO_READLINE=1):
var myrepl = require("repl").start({
terminal: false,
prompt: colors.green('% '),
ignoreUndefined: true,
useColors: true,
writer: myWriter});
var shell = require('shelljs');
Object.assign(myrepl.context, shell);
myrepl.context['inspect'] = inspect;
// add REPL command rlwrap_complete(prefix) that prints a simple list
// of completions of prefix
myrepl.context['rlwrap_complete'] = function(prefix) {
myrepl.complete(prefix, function(err,data) {
for (x of data[0]) {console.log(x);}
});
};
So this is basically what we wanted.
We have a JS repl with convenient ShellJS commands.
We also have vi keybindings, and tab completion for JS and filenames.
It’s very rough around the edges, but it was really simple to make.
GitHub user streamich
built a more advanced form
of this, called jssh
which adds many features but lacks some too.
The bottom line is, if you know JS, you might be surprised at what you can
build.