2020-Apr-12
Mainland Canada extends south to a latitude found in California
There’s a piece of France in between Nova Scotia and Newfoundland
Victoria, BC has a “warm-summer Mediterranean climate”
like Porto, Portugal and Cape Town, South Africa.
Canada’s most picturesque spot is Lake Louise
2020-Apr-1
What would a market for mathematics look like?
Formal verification might allow an elegant mechanism:
Someone posts a proposition in a formal language like Coq and the first
to submit a proof that passes verification wins the bounty.
Everything can be automated and maybe even trustless.
This has been tried, at proofmarket.org, which was shut
down
due to consistency bugs in the verifier.
Even without bugs, proof assistants are still difficult to use;
mathematician Thomas Hales says
“It is very hard to learn to use Lean
proficiently. Are you a graduate student at Stanford, CMU, or Pitt writing a
thesis on Lean? Are you a student at Imperial being guided by Kevin Buzzard?
If not, Lean might not be for you.”
If we stick to natural language to avoid the learning curve, things get messy.
How does the market decide what a complete proof is, which proof is first, and
who did it? Perhaps the only tenable solution is to leave these decisions
up to the individuals who post the bounties. How would we
know that bounties would ever get paid? Stack Exchange forces bounties to be
put in escrow and if they’re not awarded to someone there’s no refund. Another
option is to rely on reputation by using certified identities (e.g. users’
email addresses are verified and
public
so they can be checked against personal webpages).
Something along these lines might be doable (name: proofbounty.io?) but what’s
the use case?
Monetary rewards for mathematical problems are rare
and mathematicians generally already earn a salary, so the interest would
likely be modest.
Students (anywhere in the world) are plausible suppliers though, perhaps
even high school students,
while consumers could be anyone with a research grant usable for paying
“research assistants”, or industry and non-profit research groups.
A market that brings these two sides together could be of some value.
Paid question answering has been tried before, e.g.
Google Answers which
wasn’t very popular.
Did it fail due to lack of network effects,
lack of innovative mechanisms,
or an essential flaw in the concept? I don’t know.
Bounties on
GitHub issues seem to be a bit more successful.
In addition to bounties, there could be a prediction market.
The time of resolution may have to be indefinite, though, since
resolving “proposition X will be publicly proved by date Y” would in general
require determining the nonexistence of a public proof, which is at least
somewhat error-prone.
However, prediction markets are basically illegal so it’s a moot point.
2020-Mar-24
James I’s 1597 book Daemonologie,
“a philosophical dissertation on contemporary necromancy … touches on topics
such as werewolves and vampires”.
96.5% of 19-year-old males in Seoul have myopia.
List of Scottish Canadians.
Free
ebook of
classic novel plot summaries.
“Kime”: complex-valued time.
2020-Feb-17
Robin Hanson says
“In few months, China is likely to be a basket case, having crashed their
economy in failed attempt to stop COVID-19 spreading.” Quantifying the
forecast, he says China’s economy (or growth?) will be “a factor of two to ten
down” and seems to expect dramatic results in 6 months.
2020-Feb-12
Let’s analyze data from https://darksky.net from the last 10 years to compare
weather (technically “climate”) in a selection of North American cities.
If we define a “nice day” as one where
- there are at least 10 hours of daylight,
- the high apparent temperature is at least 0°C and at most 30°C,
- the cloud cover is at most 70%, and
- the UV index is at most moderate (unfortunately I used UV index at a single
point in time during the day and didn’t adjust for time zones),
we get:
City |
Probability of nice day |
San Diego |
0.27 |
Los Angeles |
0.23 |
San Francisco |
0.22 |
Raleigh |
0.22 |
Austin |
0.2 |
Vancouver |
0.19 |
New York |
0.19 |
Cambridge |
0.19 |
Chicago |
0.16 |
Ottawa |
0.16 |
Toronto |
0.15 |
What are the nicest months to visit Toronto?
Month |
Average number of nice days in Toronto |
January |
0 |
February |
2.9 |
March |
9.0 |
April |
4.7 |
May |
1.2 |
June |
0.4 |
July |
0.5 |
August |
4.0 |
September |
12.1 |
October |
15.8 |
November |
2.4 |
December |
0 |
If we define a “sunny day” as one where
- there are at least 10 hours of daylight,
- the high apparent temperature is at least 15°C, and
- the cloud cover is at most 50%,
we get:
City |
Probability of sunny day |
Los Angeles |
0.69 |
Austin |
0.56 |
San Francisco |
0.49 |
Raleigh |
0.46 |
San Diego |
0.45 |
New York |
0.33 |
Cambridge |
0.32 |
Chicago |
0.26 |
Toronto |
0.23 |
Vancouver |
0.2 |
Ottawa |
0.18 |
What are the sunniest months to visit Toronto?
Month |
Average number of sunny days in Toronto |
January |
0 |
February |
0 |
March |
0.7 |
April |
2.6 |
May |
10.0 |
June |
12.5 |
July |
17.9 |
August |
17.8 |
September |
15.1 |
October |
6.1 |
November |
0.4 |
December |
0 |
Lastly, if we define a “warm day” as one where
- the high apparent temperature is at least 15°C and at most 25°C and
- the UV index is at most high,
we get:
City |
Probability of warm day |
San Diego |
0.5 |
San Francisco |
0.45 |
Los Angeles |
0.37 |
Vancouver |
0.33 |
Raleigh |
0.28 |
New York |
0.25 |
Austin |
0.25 |
Ottawa |
0.23 |
Toronto |
0.23 |
Cambridge |
0.22 |
Chicago |
0.21 |
What are the warmest months to visit Toronto?
Month |
Average number of warm days in Toronto |
January |
0 |
February |
0.3 |
March |
1.8 |
April |
6.9 |
May |
11.7 |
June |
11.5 |
July |
4.8 |
August |
10.2 |
September |
19.9 |
October |
13.7 |
November |
2.1 |
December |
0.1 |
2020-Feb-11
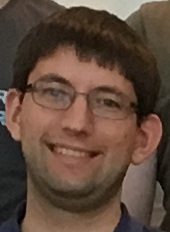
William Saunders was a fellow Fellow at MIRI in
2016 and now researches AI safety at Ought.
Below we go over his 2017 paper
“Trial without Error: Towards Safe Reinforcement Learning via Human
Intervention”.
Q:
Say we’re training an autonomous car by running a bunch of practice trips and
letting the model learn from experience. For example, to teach safe driving we
might input a reward if it makes a trip without running anyone over and input a
penalty otherwise. What’s the flaw in this approach, and how serious is this
issue in AI systems present and future?
Two big flaws, if we use traditional model-free reinforcement learning
algorithms (Deep Q learning, policy gradient):
- The RL agent won’t learn to avoid running over the human until it
actually runs over the human and recieves the penalty a large number of
times.
- The RL agent will suffer “The Sisyphean Curse of RL”. Once it learns
to avoid running over humans, it will keep having new experiences where it
doesn’t run over humans. Eventually, it will forget that running over
humans is bad, and occasionally needing to run over humans a few times and
get penalized in order to remember. This will repeat as long as the agent
is being trained.
So, the training process can lead to an arbitrary number of humans being
run over. (In practice of course, you’d stop after the first one if not
sooner).
Q:
Your proposal, called Human Intervention Reinforcement Learning (HIRL),
involves using humans to prevent unwitting AIs from taking dangerous
actions.
How does it work?
- A human watches the training process. Whenever the RL agent is about to
do something catastrophic, the human intervenes, changing the RL agent’s
action to avoid the catastrophe and giving the RL agent a penalty.
- We record all instances when the human intervenes, and train a
supervised learning algorithm (“the blocker”) to predict when the human
intervenes.
- When the blocker is able to predict when the human intervenes, we
replace the human with the blocker and continue training. Now the blocker
is called for every new action the agent takes, and decides whether it
should intervene and penalize the agent.
- Eventually, the RL agent should learn a policy that performs well on the
task and avoids proposing the blocked actions, which should then be safe
for deployment.
Q:
What’s a practical example where HIRL might be useful?
One example might be for a chatbot that occasionally proposes an offensive
reply in a conversation (e.g. Microsoft Tay). A human could review
statements proposed by the chatbot and block offensive ones being sent to
end users.
Q:
Is there a use case for HIRL in simulated learning environments?
In simulated environments, one can simply allow the catastrophic action to
happen and intervene after the fact. But depending on the simulation, it
might be more efficient for learning if catastrophic actions are blocked
(if they would end the simulation early, or cause the simulation to run for
a long time in a failed state).
Q:
In what situations would human intervention be too slow or expensive?
Even for self-driving cars, it can be difficult for a safety driver to
detect when something is going wrong and intervene in time. Other robotics
tasks might be similar.
In many domains, it might not be possible to fully hand things over to the
blocker. If the agent doesn’t try some kinds of actions or encounter some
kinds of situations until later in the training process, you either need to
have the human watch the whole time, or be able to detect when new
situations occur and bring the human back in.
Q:
How does the applicability of HIRL change (if at all) if the human is part of
the environment?
HIRL could still apply if the intervening human is part of the
environment, as long as the human supervisor is able to block any
catastrophic action that harms or manipulates the human supervisor, or the
human supervisor’s communication channel.
Q:
Theoretically the idea here is to extract, with an accuracy/cost tradeoff, a
human’s beliefs and/or preferences so an AI can make use of them. At a high
level, how big a role do you think direct human intervention will play in this
process on the road to superintelligent AI?
Ideally, you would want techniques that don’t require the human to be
watching and able to effectively intervene, it would be better if the
blocker could be trained prior to training or if the AI could detect when
it was in a novel situation and only ask for feedback then. I think
HIRL does illustrate how in many situations it’s easier to check whether an
action is safe than to specify the optimal action to perform, and this
principle might end up being used in other techniques as well.